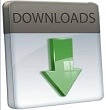
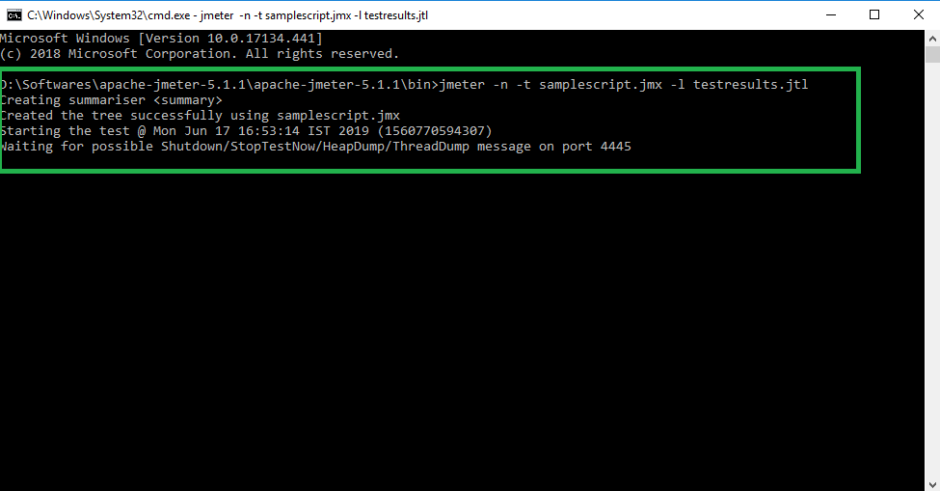
In recent years, unobtrusive or contact-free approaches have gained a lot of attention for sleep monitoring. Therefore, one would demand a contact-free sleep monitoring system for neonates. In addition, the use of VEEG is labor-intensive, where the human effort on annotating sleep states is required.
#Article text extractor skin
Ĭurrently, Video electroencephalogram (VEEG) is considered as a gold standard for neonatal sleep monitoring, which requires a number of sensors and electrodes attached to a neonate’s skin to collect multiple-channel EEG signals. To achieve this, automatic sleep and wake analysis is required, which can offer valuable information on a neonate’s mental and physical growth, not only for healthcare professionals but also for parents. Continuous sleep tracking and assessment could potentially provide an indicator of brain development over time. Normally, newborn babies sleep between 16 and 18 h per day. Sleep is an essential behavior for the development of the nervous system in neonates. Therefore, in future work a dedicated neural network trained on neonatal data or a transfer learning approach is required. This indicates that using a pre-trained model as a feature extractor could not fully suffice for highly reliable sleep and wake classification in neonates. Resultsįrom around 2-h Fluke ® video recording of seven neonates, we achieved a modest classification performance with an accuracy, sensitivity, and specificity of 65.3%, 69.8%, 61.0%, respectively with AlexNet using Fluke ® (RGB) video frames. The features are extracted after fully connected layers (FCL’s), where we compare several pre-trained CNNs, e.g., VGG16, VGG19, InceptionV3, GoogLeNet, ResNet, and AlexNet. Using pre-trained CNNs as a feature extractor would hugely reduce the effort of collecting new neonatal data for training a neural network which could be computationally expensive. The results obtained show insights related to innovative educational trends that practitioners can use to improve strategies and interventions in the education sector in a short-term future.In this paper, we propose to evaluate the use of pre-trained convolutional neural networks (CNNs) as a features extractor followed by the Principal Component Analysis (PCA) to find the best discriminant features to perform classification using support vector machine (SVM) algorithm for neonatal sleep and wake states using Fluke ® facial video frames. The results take on meaning through an application of data mining techniques and a data visualization algorithm for complex networks. The first stage employs topic-modeling using LDA (latent dirichlet allocation) to identify topics, which are then subjected to sentiment analysis (SA) using machine-learning (developed in Python).
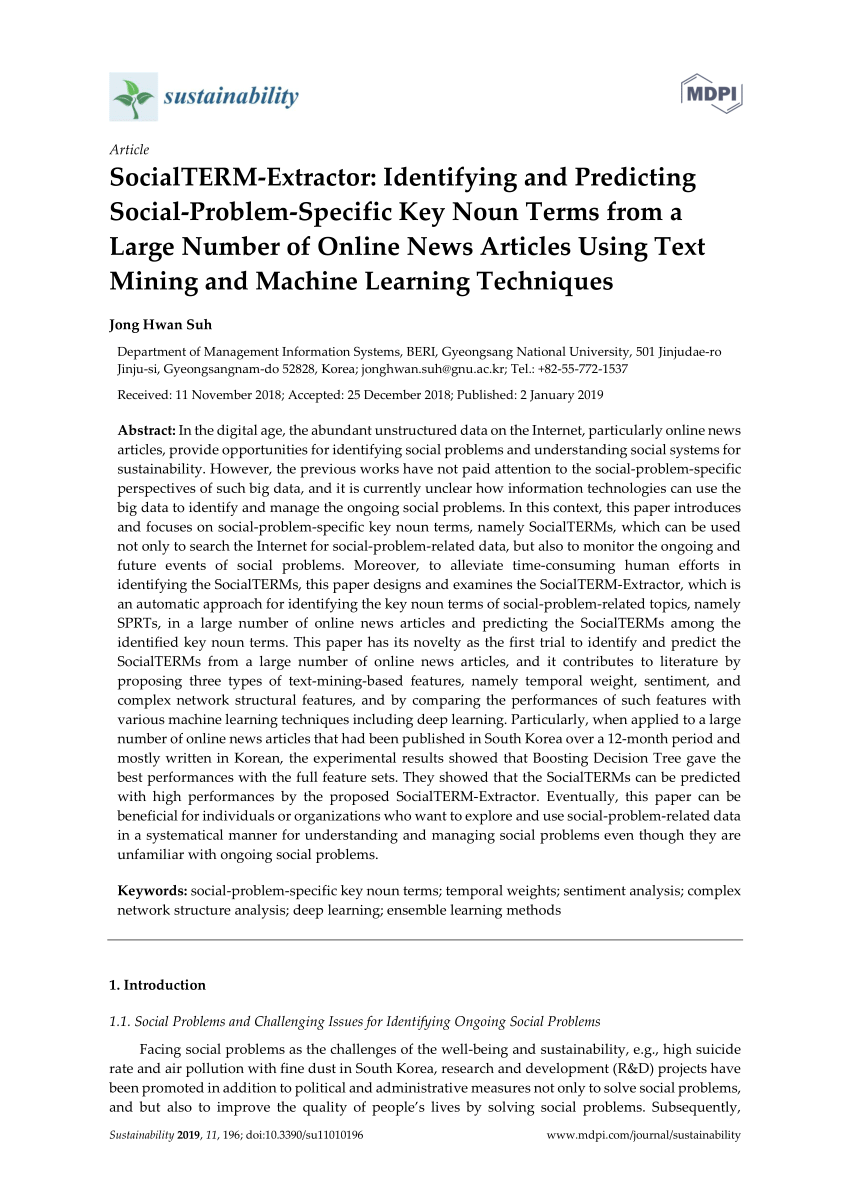
This article shows how useful knowledge can be extracted and visualized from samples of readily available UGC, in this case the text published in tweets from the social network Twitter. Students and teachers are therefore a rich source of user generated content (UGC) on social networks and digital platforms. Education is the keystone area used in this study because it is deeply affected by digital platforms as an educational medium and also because it deals mostly with digital natives who use information and communication technology (ICT) for all manner of purposes. The aim of this article is to lay a foundation for such techniques so that the age of big data may also be the age of knowledge, visualization, and understanding. New analysis and visualization techniques are required to glean useful insights from the vast amounts of data generated by new technologies and data sharing platforms.
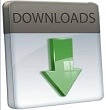